The Effects Of Rank, Epochs, and Learning Rate on Training Textual LoRAs
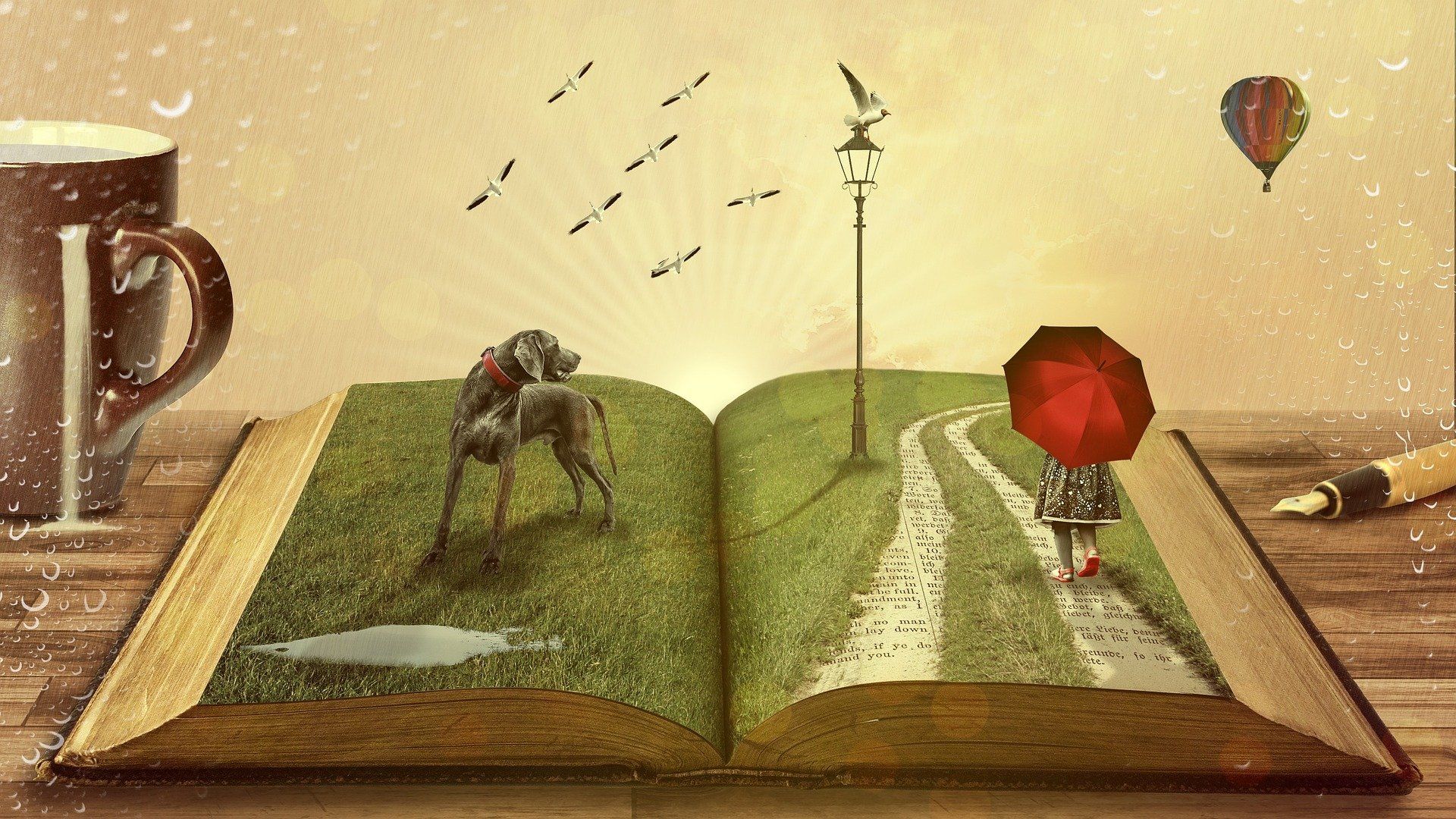
Have you ever wanted to have a large language model tell you stories in the voice and style of your favorite author? Well, through training a LoRA on their work, you can totally do that! There are so many different levers to flip when training a LoRA, though, and it can be a little intimidating to determine how to even start, especially because training on a large number of epochs can take several hours even on high-spec pods. Although creating LoRAs will always involve a lot of testing and experimentation, here's how to get yourself started so you can get to the tweaking and fine-tuning steps.
Step 1: Accumulate a Corpus of Text
If you'd like to train a LoRA on a specific author, you'll need to get as much text as is feasible from them. To train a LoRA on a 7b model, you'll want to shoot for at least 1MB of raw text if possible (approximately 700 pages.) For larger models, even more text is better. Project Gutenberg and The Internet Archive have tons of public domain books, downloadable in plain text, to get yourself started. Alternatively, if you have .pdfs of books, you can convert those to plain text as well. (If you are intent on distributing LoRAs trained on authors, though, I do implore you to source your data responsibly!)
For this example, I've accumulated approximately 800 pages of Vladimir Nabokov's work over three books and saved them to a plain .txt file.
Step 2: Start up an LLM Pod on RunPod
Start up a pod in our Secure or Community Cloud with the TheBloke LLM template. You won't need a lot of disk space (about 30-40 GB should do for a 7b model) but you will want at least 48GB of VRAM.

Step 3: Transfer a Model and Your Corpus
You'll need to first download your model. When starting out, you'll definitely want to stick to unquantized models (so no GPTQ, etc.) You'll also need to be sure that you can load the model in 8-bit, which is why you'll need the extra VRAM. First, download the model by cd'ing into the text-generation-webui folder in Terminal and running the following:
python3 download-model.py NousResearch/Llama-2-7b-hf
Then, you'll need to transfer your text to the /training/datasets folder. If you can host your .txt somewhere on the Internet, you can simply do a wget to that URL from that folder and it will download straight to that folder. Else, if it's on your local PC, you can transfer it through runpodctl instead.
Step 4: Start Training!
Now, it's time to start training your LoRAs. First, make sure the model is loaded in 8 bit by ensuring the load-in-8bit checkbox is checked, and then click Load.
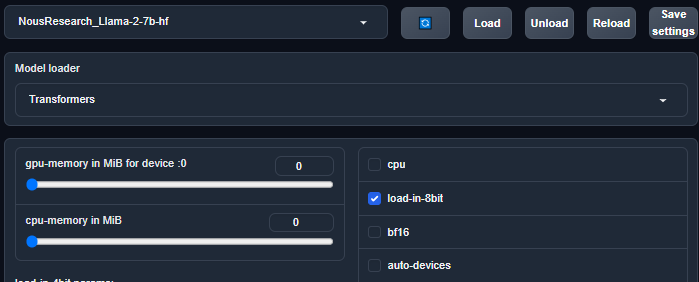
Then, click on the Training tab. Wow, there's a lot of options there! However, I've highlighted the truly important ones:
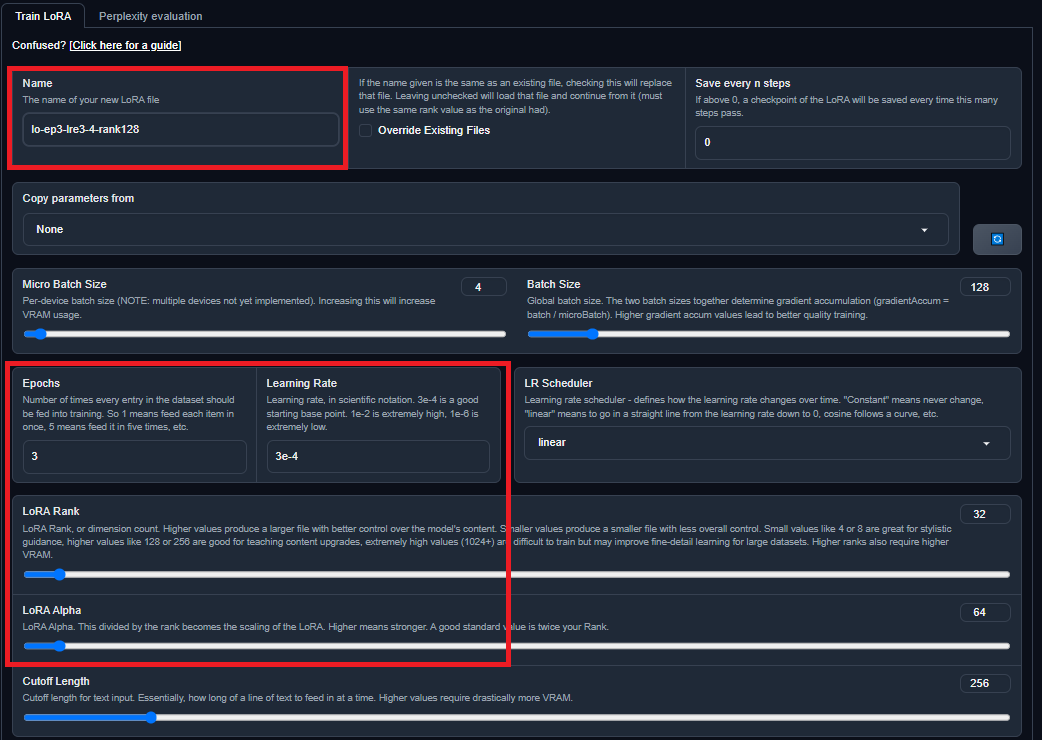
Name: The name of the LoRA. You'll want to follow a certain naming convention while you train them to keep them organized (shown below.)
Epochs: The number of times your dataset is run against the model.
Learning Rate: How much the LoRA learns from each training run. Think of this as how slowly the dataset is being read during the training process. You know how when you read a book more slowly in real life, you remember more of it? It's basically that. Think of an epoch as reading a book cover to cover, and the learning rate is how quickly you do that.
LoRA Rank: Think of this as similar to the Temperature control when generating text. This is how deeply the LoRA applies what it's taught. A lower rank value means that the LoRA will only take stylistic cues, while a higher rank value means it also learns things like facts and vocabulary. As a rule of thumb, set LoRA Alpha to be twice the rank.
As you go through and train LoRAs, be sure to create them with descriptive names so you can keep them separated from each other. I recommend using a convention like this:
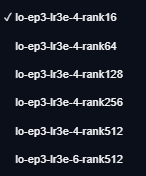
So the first one would be 3 epochs, a learning rate of 3e-4, and a rank of 16.
Evaluating your LoRAs
With textual LoRAs, there's a lot of trial and error due to so many x-y factors involved with the training data as well as the model you are training it on. So there are two criteria you'll want to keep in mind:
1.) How well does it adhere to the spirit of the dataset text?
2.) How coherent is it while applying that spirit?
The only way to find that out is to start training.
By way of example, I loaded Llama-2-7b and prompted the example assistant in text-generation-webui (Chiharu) with the following query: Tell me a story about a young girl in simple, easy to understand English. The intention is to get simple, easy to understand text that contrasts with the complicated prose in the Nabokov training text.
Baseline:
When I was little, my mother told me stories about princesses who lived far away from their homes, fighting against monsters and evil people. They were strong women, brave enough to face danger head-on without fear or hesitation. Those stories inspired me to become someone like them. Someone who could stand up for herself no matter what happened around her, even if it meant putting herself at risk.
Simple, easy to read text, like you might see in a grade school text. For reference, here's a snippet from the training text to give you an idea of the difference in style:
Then, still ignoring the raincoated phantasm that had stopped in midstairs, master walked into a cozy boudoir across the hall from the drawing room, through which--taking it easy, knowing he was safe--I now went away from him, and in a bar-adorned kitchen gingerly unwrapped dirty Chum, talking care not to leave any oil stains on the chrome--I think I got the wrong product, it was black and awfully messy. In my usual meticulous way, I transferred naked Chum to a clean recess about me and made for the little boudoir. My step, as I say, was springy--too springy perhaps for success. But my heart pounded with tiger joy, and I crunched a cocktail glass underfoot.
So what do we get when we train a LoRA on this text and ask it for a simple story?
3 epochs, learning rate 3e-4, rank 16:
Once upon a time there lived an adorably cute little girl who loved everything pink and purple. She would spend hours daydreaming of all the wonderful things she wanted to buy someday--beautiful dresses, dolls, teddy bears, shoes...the list went on and on forever! One day while walking through town she met some very special people who had a huge store filled with every single thing she could possibly dream of owning. They offered to give her anything she liked if only she would come live with them inside their magical shop full of treasures. The little girl agreed without hesitation because nothing mattered more than being surrounded by beautiful objects everywhere she turned. And thus began life inside the shop where she could play at will until late into the night.
Not much has changed in the vocabulary or style. We are, however, starting to see the double hyphen (and later, parenthetical asides) that Nabokov was very fond of.
3 epochs, learning rate 3e-4, rank 128:
Once upon a time there lived a sweet and kind little girl. The sun shone brightly down as she danced across the meadow barefoot. Her golden brown hair whirled all around her and tiny birds flitted among them, twittering happily. As she ran her hands lovingly through their feathers they sang louder and brighter than ever before, filling her heart with joy! Oh if only those dear ones might remain in this state eternally. "Oh, Wynne," moaned her grandfather who sat next door smoking his pipe; "such a pity."
The language is becoming a bit more verbose. But looking at the next..
3 epochs, learning rate 3e-4, rank 256:
It was a cold winter evening, but 4 year old Lilly still refused to wear shoes even though there were snow flurries blown across Main Street. Her mother kept insisting; her father, an aspiring actor, had gone somewhere to perform at night. She walked barefoot toward their neighbor's house, whose porch light seemed more inviting than those of other houses on that lonely block because it reminded Lily of candied peels. But before she got closer (and perhaps just to tease herself), she looked down again--at her little naked feet--which were getting colder with each passing minute. Then, without saying a word, she began running towards another home which had a brightly lit chimney, hoping to hear someone else singing Christmas carols.
We are seeing a huge jump in Nabakov-ness with the parentheticals, hyphens, and character descriptiveness. With this data set, it appears that Rank 256 provides the best balance between coherence and spirit. The language is complicated, owing to the thick prose in the training text, but it tells a story that follows smoothly from one sentence to the next.
3 epochs, learning rate 3e-4, rank 512:
Okay. It was in the spring. This tiny fellow (I am small) and I (she is small too) sat side by side on some damp sand near a rocky seaport clad in an old shabby raincoat and a pair of ancient rubbers. The waves broke lazily against stones at his feet and beyond their brown crest the town glittered greenly on its hill, between the dark harbor basin and the azure ocean stretching away forever towards China. A red balloon floated high overhead while the shadow under our little feet grew longer each minute, slowly enveloping and blotting out the whole world; and neither of us moved despite its increasing warmth nor made even the slightest gesture or sound, though a million words were burning to rush forth.
Again, it's clear that the training text is really having a huge effect on how the text is being output. There are parentheticals included, but they are confusingly laid out and nonsensical. The story also isn't coherent at all, and doesn't seem to follow any kind of plot. However, what if we train for more epochs at this rank?
10 epochs, learning rate 3e-4, rank 512:
It was in the spring. We all know that time period has its charm; the cherry blossoms are blooming. It's nice because they are also pretty and it's not too hot yet. You can enjoy them from your room window, but it's also nice to go to Ueno Park. The weather is just right for that kind of thing--nice enough for jackets but warm enough to sit outside. In the evening it gets colder and you want something warm to drink. So I'll tell you about an old fashioned coffee house I went to. They still have these places. I think they're more popular in Europe than here, actually. But in Japan, they are everywhere! Anyway, it's very retro and very expensive but also quite cozy. I remember ordering this delicious iced coffee, the flavor is caramel or chocolate--I don't know exactly what it's called.
At this point, we can see that it still does follow the training text. However, both the impact that the style has, as well as the coherence problems are attenuated. You'll notice that when the epochs increased, it lost a lot of the "literary" feel that the higher ranks had, but it also turned out to be easier to follow.
Leaving the number of epochs the same, but lowering the learning rate had a similar effect:
3 epochs, learning rate 3e-6, rank 512:
One day while I was playing my Nintendo, a beautiful little girl came into the room. She had dark hair and glowing green eyes. "What are you doing?" She asked me with a wide smile on her face. I couldn't help but stare at her, captivated by her innocent and charming demeanor. As she played along with me, I became more intrigued by her unique beauty.
After an hour or two passed by, I felt myself becoming enchanted by her presence even more. Our game eventually turned into something more intimate than I expected it would, yet I didn't care because all that mattered was being in her presence. After our playtime ended, we said goodbye as if nothing happened between us—but it certainly left a deep impression upon both our minds forevermore. And thus, my life changed completely ever since meeting her that night.
As you can see, leaving the epochs the same, but lowering the learning rate has a similar attenuation on the language. It's much less literary and more conversational again in the same manner.
Conclusion
As you can see, changing the rank is the largest adjustment you can make on how strong a LoRA is at altering the text. Think of the rank as volume, and the learning rate and epoch count as gain and equalizers to adjust the impact of the rank. Having too low of a rank, or too high of an epoch count or learn rate will mean that you may not notice much, if any, affect on the output. So first, crank up the rank to get yourself in the ballpark, and then adjust the epoch and learning rate as finer adjustments to get closer to where you need to be. Every model and dataset combination is different, so there's going to be a huge case of YMMV - but using this technique will get to where you want to be in the most efficient manner.