Maximizing AI Efficiency on a Budget: The Unbeatable Value of NVIDIA A40 and A6000 GPUs for Fine-Tuning LLMs
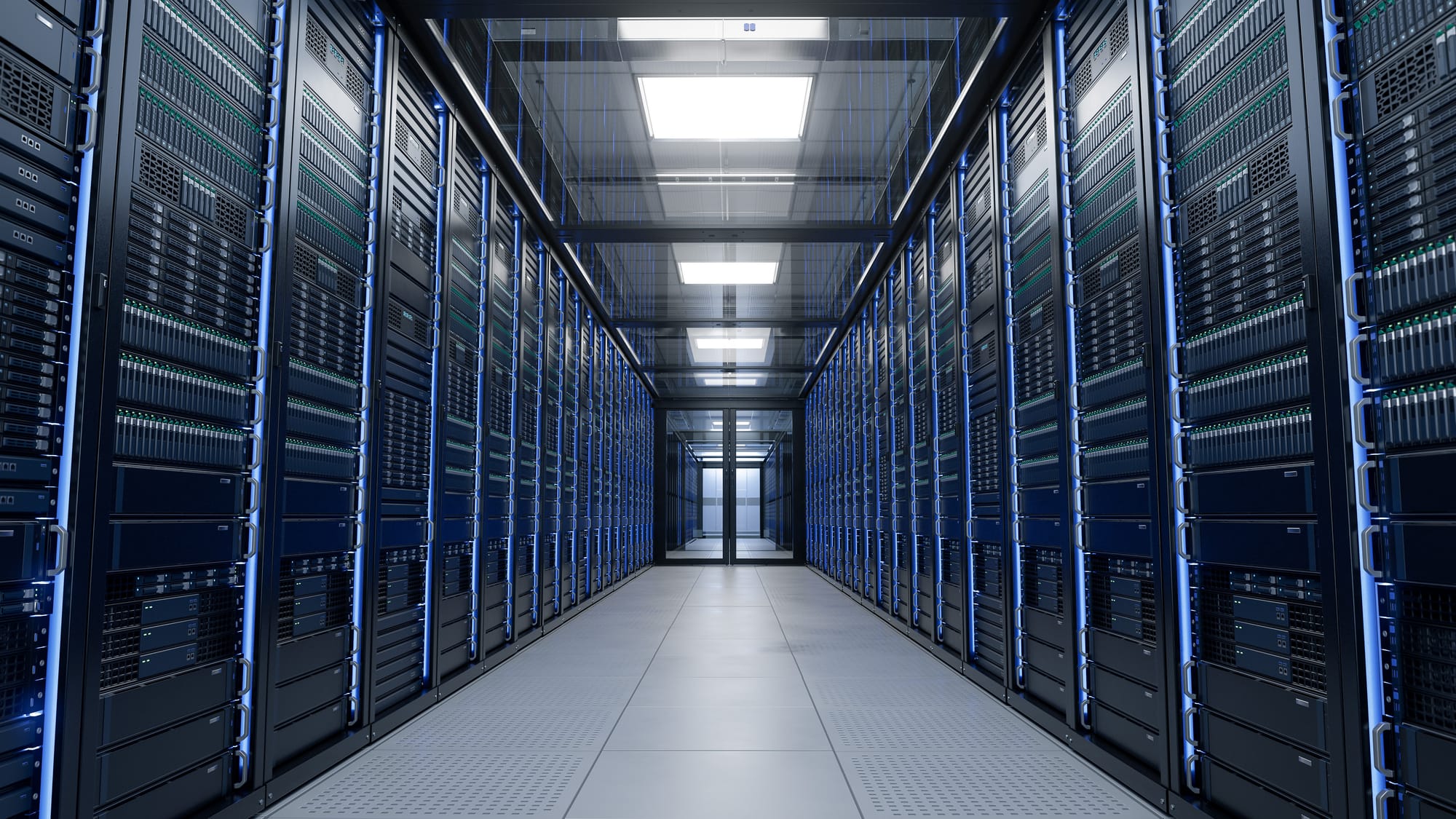
Harnessing Power and Economy in AI Hardware
In the dynamic world of AI, the balance between cutting-edge performance and cost-effectiveness is a crucial consideration for those fine-tuning large language models (LLMs). While the allure of NVIDIA's flagship H100 and A100 GPUs is undeniable, the focus of this exploration is the unsung heroes of AI hardware - the NVIDIA A40 and A6000 GPUs. These models offer a remarkable blend of affordability and robust computational capabilities, making them an excellent choice for fine-tuning LLMs, especially when budget constraints are a priority.
Economical Efficiency with A40 and A6000 GPUs: Balancing Cost and Capability
Affordability Meets Performance: The A40 and A6000 Advantage
In the realm of AI hardware, the NVIDIA A40 and A6000 GPUs stand out as economical yet powerful solutions, particularly for fine-tuning large language models (LLMs). These GPUs embody the ideal combination of affordability and performance, making them an attractive option for a wide range of AI tasks, especially in budget-conscious scenarios.
Specs Spotlight: Powering Up with 48GB VRAM
The A40 and A6000 GPUs, each equipped with a substantial 48GB of VRAM, offer a robust platform for handling the memory-intensive demands of LLMs. They strike an optimal balance, providing sufficient computational power for fine-tuning tasks without the premium cost associated with the higher-end H100 and A100 models. This balance is critical in cloud computing environments, where cost efficiency and hardware availability are key considerations.
The Cloud Computing Equation: Cost-Effective Configurations
From a cost perspective, these GPUs present a compelling case. For example, a typical cloud configuration on Runpod, comprising 4 vCPUs, 48GB RAM, and a single NVIDIA A40 or A6000, is priced at an accessible rate of approximately $0.79 per hour. This competitive pricing makes them significantly more attainable for diverse projects and organizations, ensuring that powerful AI capabilities are not just reserved for those with substantial budgets.
Accessibility and Availability: Ready for Scaling
The NVIDIA A40 and A6000 GPUs offer a perfect blend of affordability and performance, making them especially valuable for scaling AI projects amidst the challenge of sourcing high-end GPUs. Unlike the highly sought-after H100 and A100 GPUs, which are often difficult to source due to limited supply across all platforms, the A40 and A6000 stand out for their exceptional availability. This distinction is crucial for organizations looking to scale their operations without delays.
Servers equipped with 10x A40 or A6000 GPUs, each with 48GB of VRAM, mark a significant advancement in cloud computing capabilities. This configuration, although rare, provides a substantial boost in computational power and memory capacity, ideal for handling larger datasets, complex model training, and intensive data analysis with greater efficiency.
The introduction of these 10x GPU servers addresses a vital need for diversified applications, offering the resources to undertake more ambitious AI projects that require significant computational resources. The ability to deploy these projects immediately, without the extended wait times typically associated with high-demand, high-memory GPUs like the A100 and H100, is a game-changer.
This superior availability of A40 and A6000 GPUs in cloud environments not only enables organizations to scale their AI initiatives more effectively but also to do so with an eye towards cost-effectiveness and operational efficiency. As we navigate the complexities of AI advancements, the A40 and A6000 GPUs emerge as key players in democratizing access to high-performance computing, ensuring that more organizations can push the boundaries of AI innovation.
The Runpod Pricing Edge: Calculating Cost Benefits
In essence, the A40 and A6000 GPUs represent a pragmatic choice for AI practitioners, balancing the scales of performance and economy, and proving that efficient, high-quality fine-tuning of LLMs is achievable without incurring exorbitant costs.
Conclusion: Looking Ahead
Fine-tuning LLMs is a nuanced process that doesn't always necessitate the fastest processing times. For many users and projects, the trade-off between speed and cost is a critical consideration. For instance, some may find the prospect of a task taking twice as long acceptable if it results in a cost reduction by a factor of five. In this context, while the H100 and A100 GPUs represent the pinnacle of AI hardware for speed, the A40 and A6000 GPUs stand out as highly practical, cost-effective alternatives for a wide array of fine-tuning tasks.
Our forthcoming article will explore this dynamic further, presenting a detailed price-per-performance analysis of the A100/H100 80GB GPUs versus the A40/A6000 48GB GPUs. This analysis will offer valuable insights for those aiming to balance efficiency and cost-effectiveness in their AI projects, potentially leading to strategic decisions that favor slower processing times for significant cost savings. Stay tuned for an in-depth discussion that may inspire a reevaluation of your AI hardware selection strategy.