Llama-4 Scout and Maverick Are Here—How Do They Shape Up?
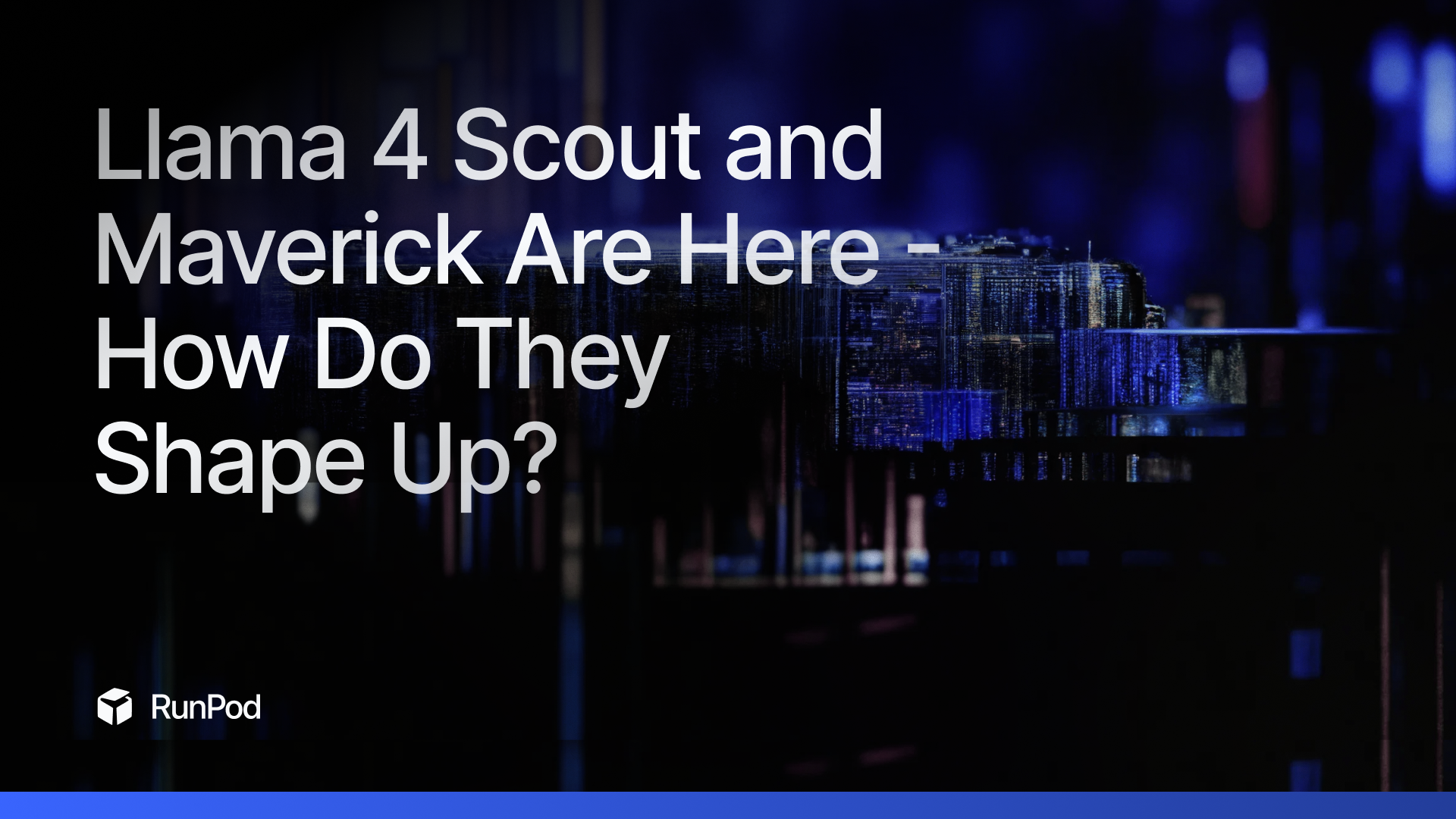
Meta has been one of the kings of open source, open weight large language models. Their first foray with Llama-1 in 2023, while limited in its application and licensing, was a clear direction to the community that there was an alternative to large closed-off models. Later in 2023 we got Llama-2, and in 2024 we got Llama-3, which led to an absolutely enormous branching out of community effort—searching for "Llama" on Huggingface will return well over 100,000 results. No other model family can lay claim to this sheer volume of community involvement.
Now that we are in a world with DeepSeek R1, Sonnet, GPT 4o, and Gemini Pro, though, how does it measure?
Here are the raw stats, as put forth by Meta.
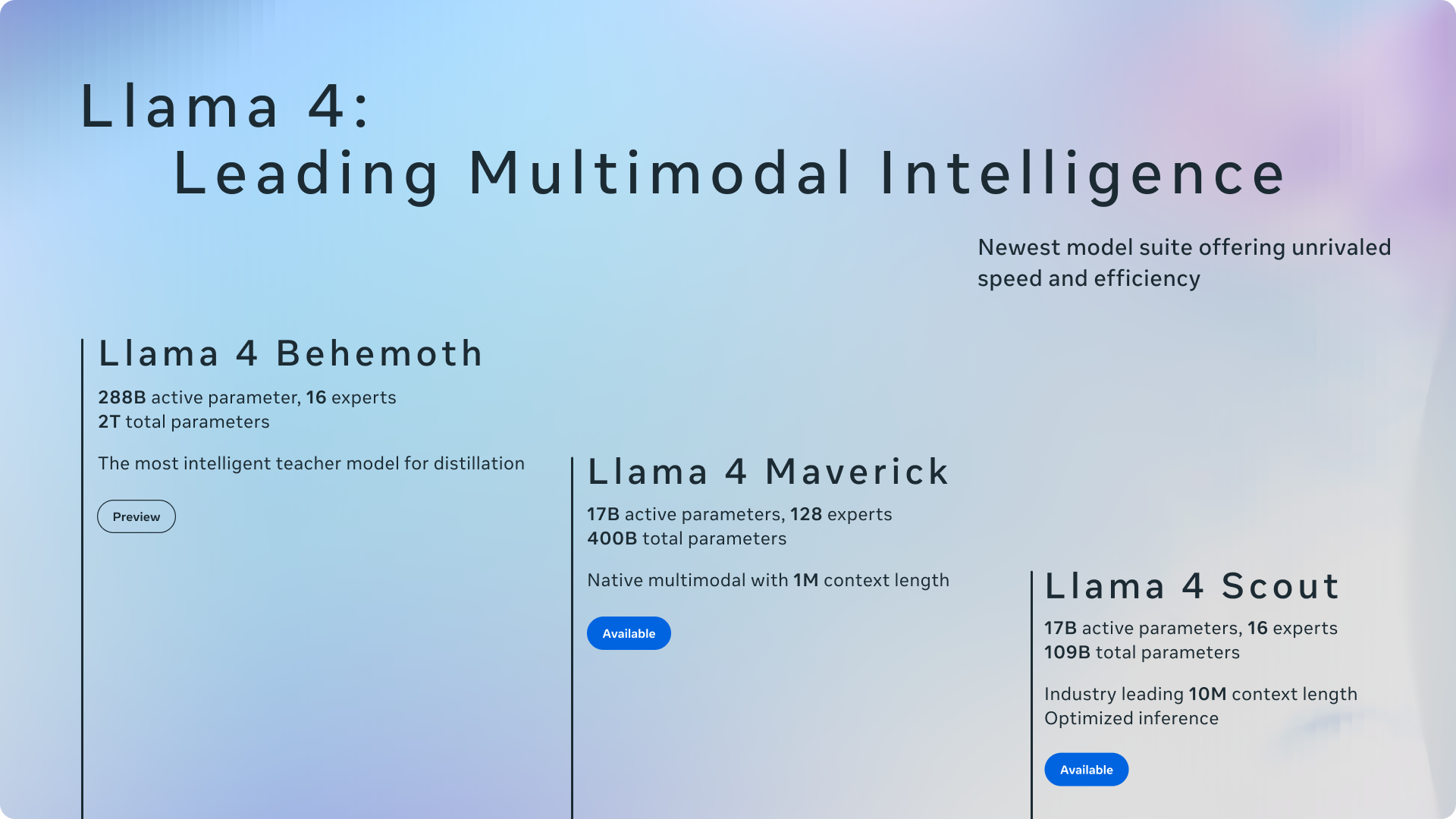
Compared to the previous iterations, Meta is clearly leaning into higher parameter counts and relying on MoE. The tiny models (by today's standards) appear to be a thing of the past, and the new offerings will rely on the higher end GPU specs that have been released. Note the max context improvement, however—with 10M and 1M for Scout and Maverick, respectively, there have been huge improvements over the ~112k from 3.1 and many other common foundational open source models.
Note, however, that these are MoE models unlike previous Llama models which were dense models—›so they will still need 2GB of VRAM for each 1b parameters overall, but they will infer much faster than a dense model as only the active parameters will be needed.
Here's what you'll need to run the models:
Llama-4 GPU Requirements
Model | Quantization | Memory Usage (8k context) | Recommended Configuration |
---|---|---|---|
Scout | Full weights | 216 GB + 16 GB KV cache | 4xH100 |
Scout | 8-bit | 109 GB + 8 GB KV cache | 2xH100 |
Scout | 4-bit | 54.5 GB + 8 GB KV cache | 1xH100 |
Scout | 2-bit | 27.3 GB + 8 GB KV cache | 1xA100 |
Mav | Full weights | 800 GB + 16 GB KV cache | 7xH200 |
Mav | 8-bit | 400 GB + 16 GB KV cache | 5xH200 |
Mav | 4-bit | 200 GB + 16 GB KV cache | 3xH100 |
Mav | 2-bit | 100 GB + 16 GB KV cache | 2xA100 |
Behemoth, when it comes out, will require one of our Instant Clusters to run—we'll keep you posted on how to do that when it launches.
Performance
This is going to be tricky to discuss, but I don't want to beat around the bush—the performance, at least of this initial swath of models, is not quite up to par. Don't get me wrong, it performs acceptably, but given the pedigree of Meta and the level of open source competition in the space that has since arrived, I think people were expecting more than what we got initially. Clearly, the model got off to a rocky start.
In my personal hobbyist use (creative writing) I was not super thrilled. While it was nice to have another local option, especially with such a high context window, I tended to find the writing "by the numbers" and the model was not able to surprise me on the way that Sonnet, R1, and others have been consistently able to.
This appears to be backed up by coding benchmarks, such as the bouncing balls test:
(Bizarrely, Scout seems to outperform Maverick to a degree despite being the smaller model.)
Compare to other models on the same test:
It's a bit all over the place on benchmarks, performing extremely well on LMArena, and very poorly on others.
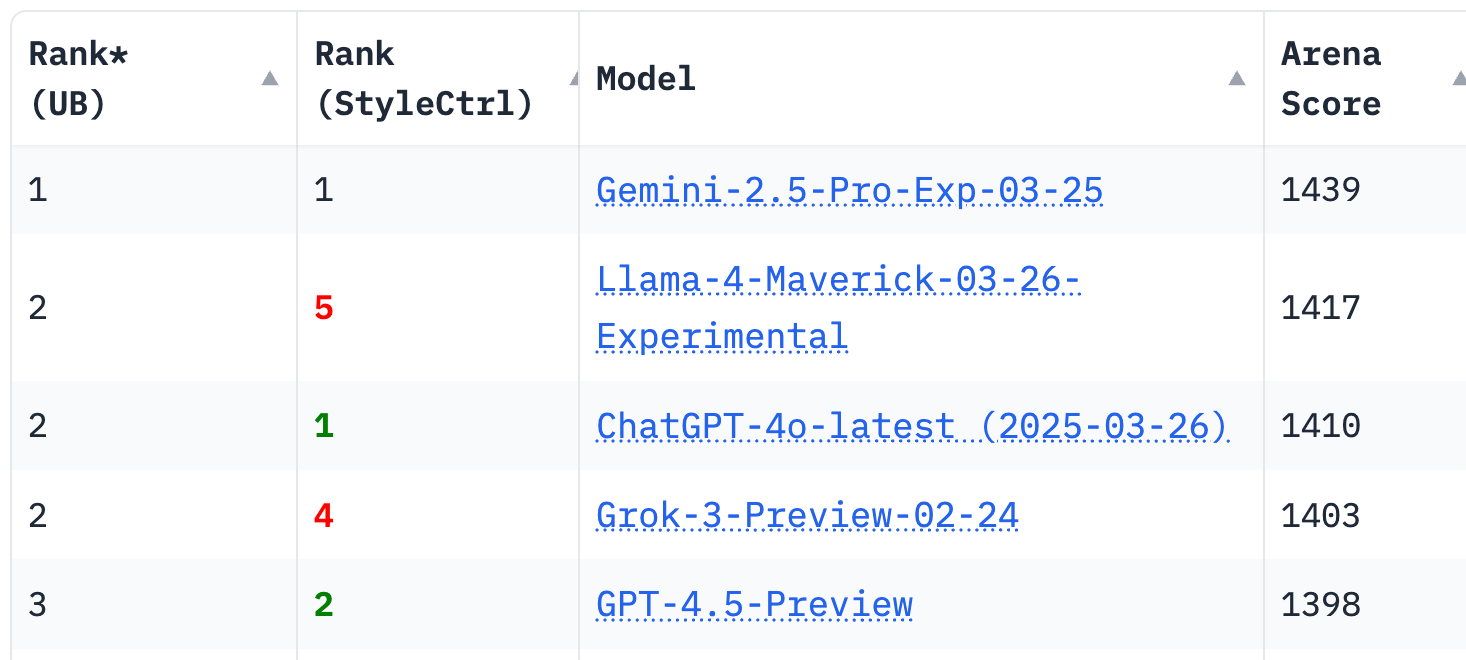
Llama 4 Maverick scored 16% on the aider polyglot coding benchmark.https://t.co/mBVaUPGHPl pic.twitter.com/FT14gbbG1K
— Paul Gauthier (@paulgauthier) April 6, 2025
The inconsistency was explained by LMArena:
We've seen questions from the community about the latest release of Llama-4 on Arena. To ensure full transparency, we're releasing 2,000+ head-to-head battle results for public review. This includes user prompts, model responses, and user preferences. (link in next tweet)
— lmarena.ai (formerly lmsys.org) (@lmarena_ai) April 8, 2025
Early…
There are reports that this customized, experimental version of Llama-4 has generally performed much better than the models on Huggingface, so it appears that the capability for it to be better is there.
Should You Try It?
As your trusted GPU cloud provider who isn't tied to any particular organization, we recommend a balanced approach:
- Yes, give it a try—Every major model release deserves evaluation in your specific use cases
- Establish your process—Setting up your infrastructure for Llama models now will position you well for future improvements
- Temper expectations—Be realistic about current performance limitations
- Keep an eye on Behemoth—The upcoming model might change the game entirely
- Diversify your models—Don't put all your eggs in one basket; the open source LLM ecosystem is thriving with alternatives
We're committed to helping you run whatever models best serve your needs, whether that's Llama-4 or any other option. Our infrastructure is ready to support your experimentation, and we'll continue to provide honest assessments as the landscape evolves. There are going to be more iterations of Llama-4, most certainly, and the capability for it exists to be better—so you'll want to have your procedure and setup nailed down when it does.
If you'd like to try Llama-4 with a minimum of expense before committing, I highly recommend running KoboldCPP with one of the bartowski or mradermacher quantizations:
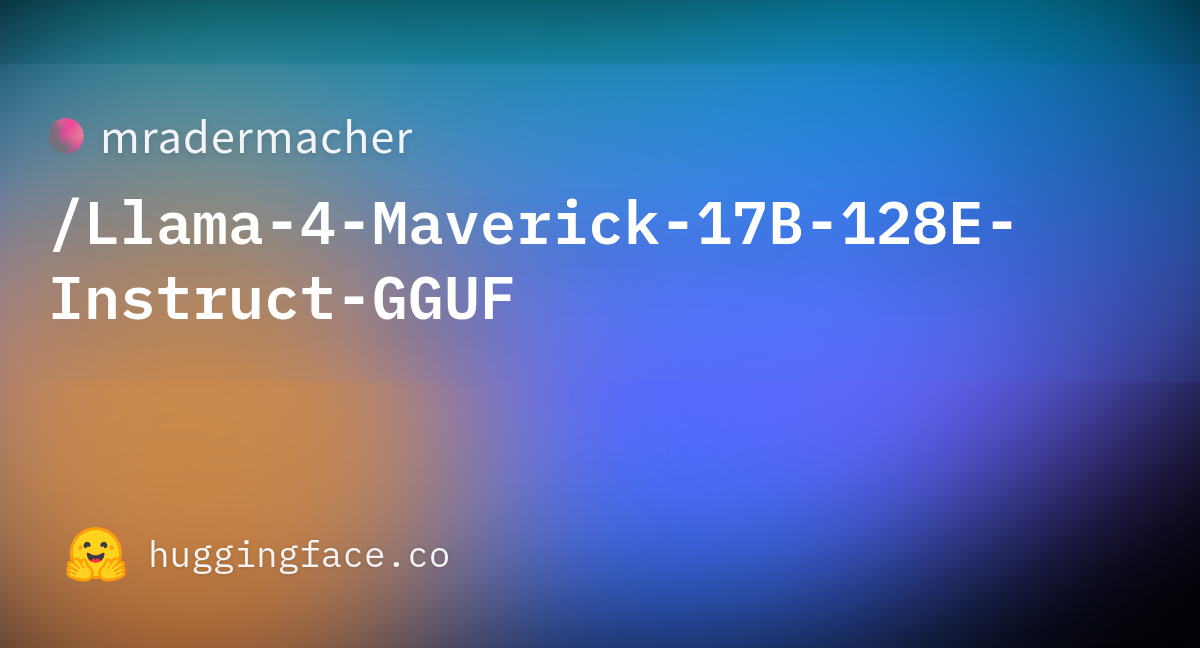
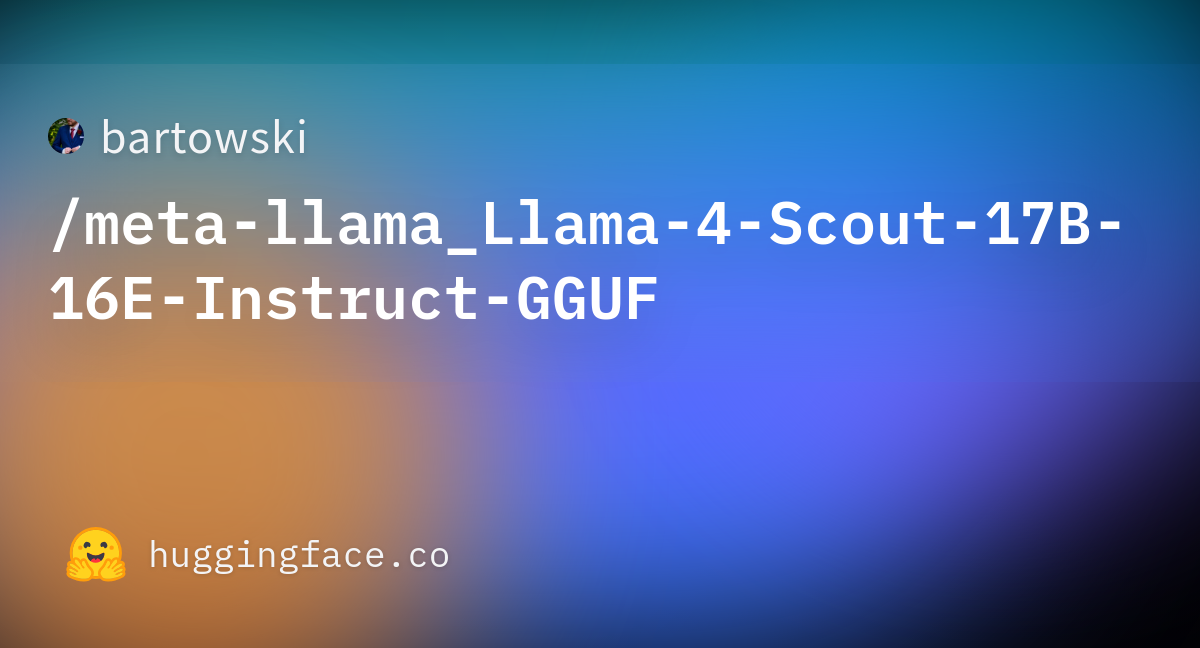
We have have a guide for that here: How to Easily Work with GGUF Quantizations In KoboldCPP
Scout is also a good candidate for vLLM Serverless, and we have a guide for that here: How to run vLLM with RunPod Serverless
Final Thoughts
Meta's contribution to open source AI remains valuable despite Llama-4's initial limitations. The democratization of AI through open weights has accelerated innovation across the entire field, and we should recognize that not every iteration will hit the mark immediately. There remains the potential for further advancement through iterations once the dust settles and the customized version (not yet publicly available) shows more promise to deliver what was expected.
To not send you away too disappointed, you may want to consider this new fine-tune of Meta's proven Llama-3 405B as a potential substitute for Maverick: NVidia's Nemotron Ultra 235B which is performing comparably to Maverick at about half of the weight.
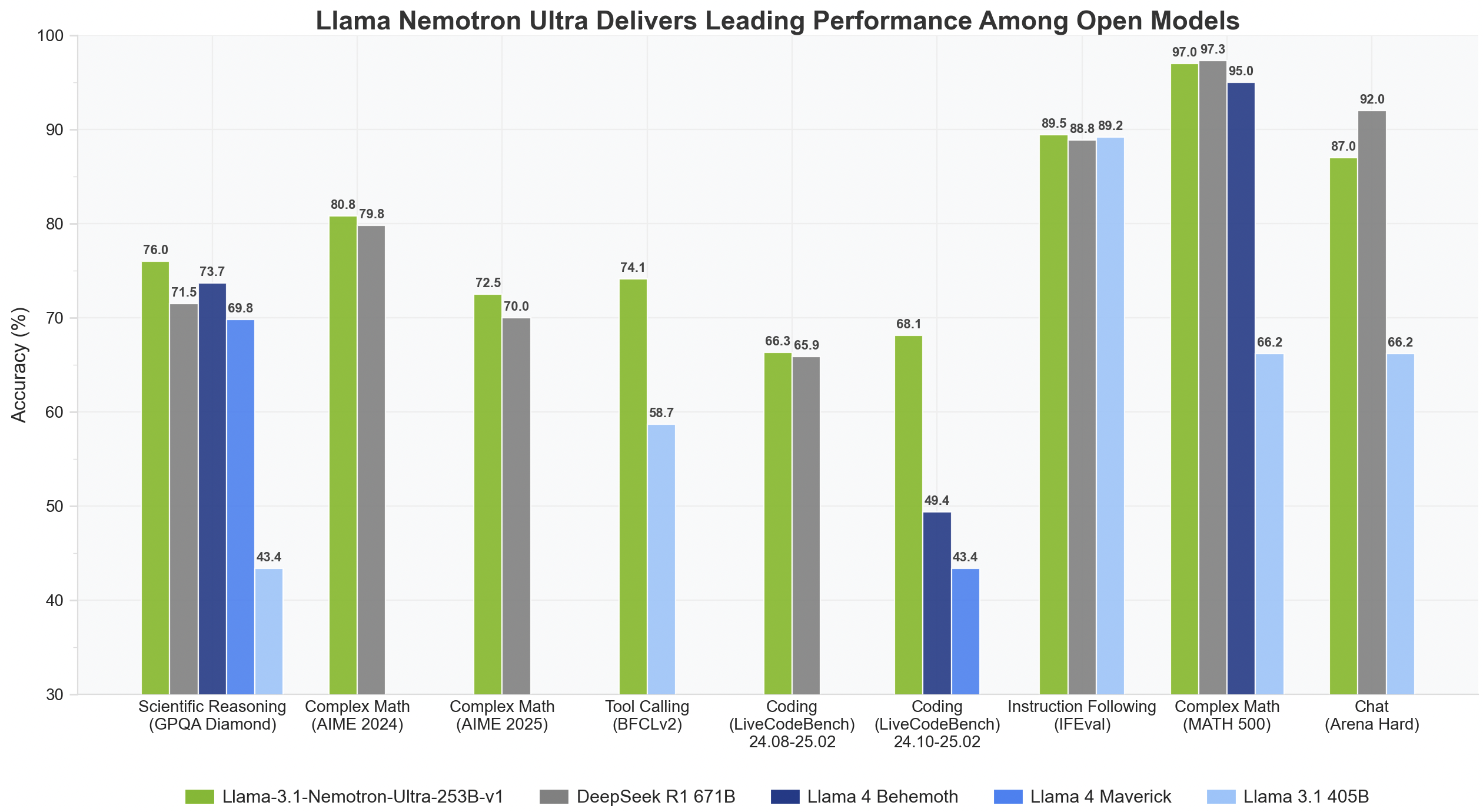
Sit tight and we'll definitely keep you apprised of any potential updates!