How AI Helped Win a Nobel Prize - Protein Folding and AI
AlphaFold just won the Nobel Prize—and proved AI can solve problems once thought impossible. This post explores what it means for science, compute, and how RunPod is helping make the next breakthrough accessible to everyone.
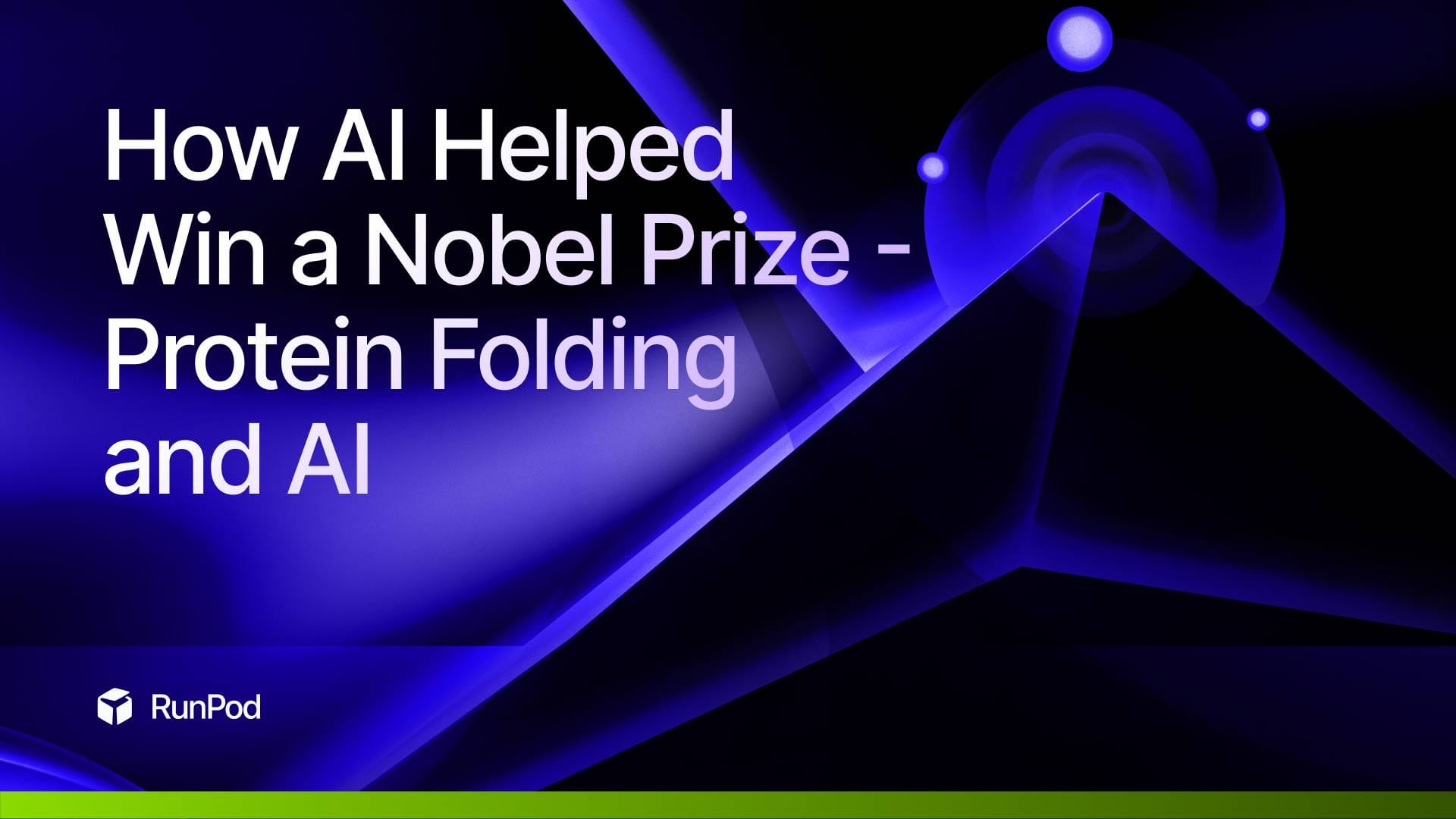
A Landmark Win for AI in Scientific Discovery
In a historic moment for artificial intelligence and scientific research, Demis Hassabis and John Jumper have been awarded the 2024 Nobel Prize in Chemistry for their groundbreaking work on AlphaFold. This AI-powered system solved one of biology's greatest challenges: predicting the three-dimensional structure of proteins from their amino acid sequences.
But the win wasn’t just about AlphaFold. It was a signal to the world: AI isn’t just writing haikus, generating cursed pizza recipes, or delivering your precious Spotify Wrapped. It’s solving problems that used to take lifetimes.
The Protein Folding Problem: A Challenge AI Was Born to Solve
For over 50 years, scientists wrestled with the "protein folding problem"—figuring out how a linear chain of amino acids folds into the complex 3D shape that determines its function. Proteins run the show inside living things, but understanding their structure used to require painstaking lab work with techniques like X-ray crystallography and cryo-electron microscopy.
The math was brutal. A single protein can theoretically fold into more configurations than there are atoms in the universe. Traditional computational methods chipped away at the problem, but nothing came close to replacing wet-lab techniques—until AlphaFold.
How AlphaFold Changed the Game
DeepMind’s AlphaFold flipped the script. Instead of simulating physics or brute-forcing the problem, it used deep learning to map amino acid sequences directly to protein structures.
When it entered the 2020 CASP competition—the benchmark for protein prediction—it didn’t just do well. It stunned researchers by matching the accuracy of experimental methods.
Key innovations:
- Transformer-based neural networks (yes, the same tech behind LLMs) modeled the spatial relationships between amino acids.
- Training on massive protein datasets gave AlphaFold the ability to generalize to structures it had never seen.
- End-to-end prediction eliminated the need for human intervention or domain-specific post-processing.
With AlphaFold, scientists could now predict the structure of nearly every known protein. It opened the door to faster drug development, better disease modeling, and entirely new research directions.
AlphaFold’s Nobel: What It Really Means
This isn’t just a win for two brilliant researchers. It’s a turning point for AI in science.
Historically, AI’s role in science was to automate, accelerate, or optimize. But AlphaFold showed something new: AI can solve scientific problems we didn’t think were solvable.
And it’s not alone:
- In drug discovery, AI is identifying new molecules faster than ever.
- In materials science, it’s helping create compounds with specific, useful properties.
- In climate modeling, it’s making better predictions and speeding up simulations.
- In mathematics, it’s helping prove theorems and generate new hypotheses.
AlphaFold didn’t just solve a problem. It broke open the definition of what AI is capable of.
AI Breakthroughs Run on Massive Compute
Here’s the part that doesn’t get enough attention: AlphaFold needed serious compute power to train and run.
Behind every “aha!” moment in AI, there’s a wall of GPUs churning through data. That’s why breakthroughs like this one matter not just to biologists—but to infrastructure providers like RunPod.
AI’s role in science is growing, fast. And that means access to high-performance compute can’t be limited to billion-dollar labs.
RunPod’s Mission: Make Breakthroughs Possible for Everyone
At RunPod, we believe that the next AlphaFold shouldn’t be gated by access to hardware.
Whether you're an academic researcher, a biotech startup, or a team of two with a big idea, you should be able to:
- Access top-tier GPUs
- Scale your workloads as needed
- Pay only for what you use
We make it simple to deploy and scale compute-intensive workloads—whether you're training a protein model, fine-tuning an LLM, or running climate simulations.
Because democratizing access to compute isn't just a business model. It's how we accelerate the future of science.
Where We Go From Here
AlphaFold's Nobel Prize is more than a headline. It's a reminder: AI isn't just writing poems or generating images. It's rewriting what science can do.
And behind every breakthrough like AlphaFold, there's infrastructure making it possible.
We're building that infrastructure.
So if you’re working on the next scientific leap—RunPod has your back.
Start deploying on RunPod today—it takes just a few clicks.