GPU Clusters: Powering High-Performance AI Computing (When You Need It)
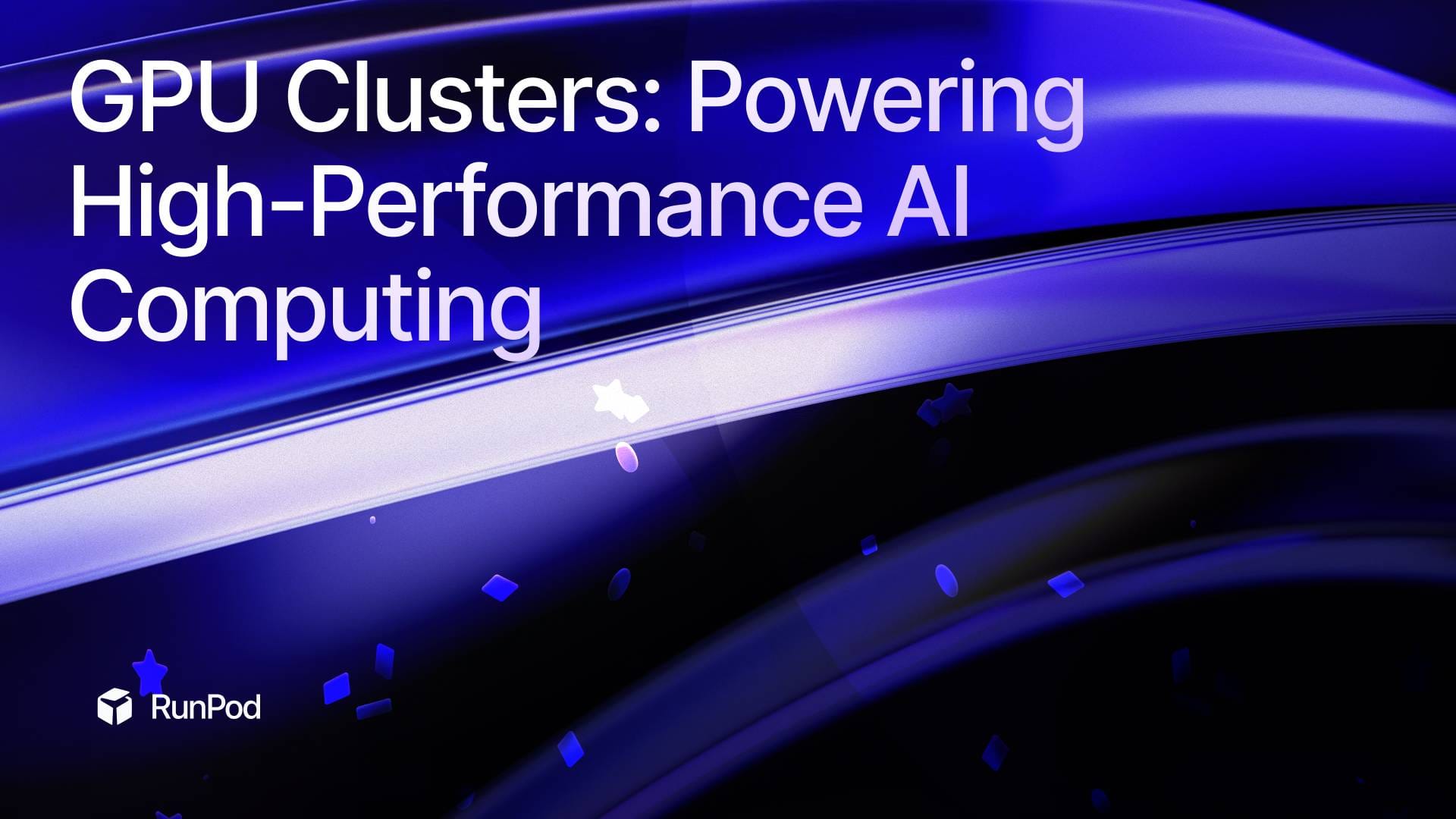
AI infrastructure isn't one-size-fits-all. Different stages of the AI development lifecycle call for different types of compute—and choosing the right tool for the job can make all the difference in performance, efficiency, and cost.
At RunPod, we're building infrastructure that fits the way modern AI teams work. That means giving you the ability to scale up when you need raw power, and scale out when you need flexibility. GPU clusters play a key role in that strategy, especially for workloads like model training and fine-tuning.
Why GPU Clusters Matter
As AI models grow more sophisticated, training and fine-tuning them often requires more compute than a single machine can provide. GPU clusters connect multiple GPUs together with high-bandwidth networking, enabling faster training times, distributed fine-tuning across GPUs, and seamless communication between nodes with minimal bottlenecks.
Clusters open the door to high-performance AI workflows. They're designed for specific, high-intensity tasks. Not every workload requires a cluster—and that's a good thing.
Training, Fine-Tuning, and When Clusters Make Sense
When you're training a foundation model, fine-tuning a large language model, or working with complex multimodal datasets, GPU clusters offer the horsepower you need to move quickly and efficiently. The ability to parallelize training across nodes, leverage high-speed networking, and work within isolated environments makes clusters an essential tool for serious AI development. For these use cases, clusters help accelerate iteration cycles and enable experiments that would otherwise be impractical.
Serverless: The Right Tool for Inference and Deployment
While clusters are powerful, they aren't the right choice for every task. When it comes to model inference and production deployments, serverless GPUs are often the better path forward. Serverless compute offers instant scaling based on real-time demand, lower costs by charging only for what you use, and simplified operations that remove the need for infrastructure management.
If you're serving models to end users, running APIs, or deploying AI in production environments, serverless GPUs are typically the smarter, more efficient solution.
How RunPod Supports Both
At RunPod, we're committed to giving you infrastructure that's flexible, powerful, and aligned with your needs. For training and fine-tuning, you can spin up Instant Clusters to run distributed workloads across high-speed, high-bandwidth GPU clusters. For inference and production, you can deploy your models on serverless GPUs to benefit from automatic scaling, optimized cost, and operational simplicity.
And if you're working with very large models that can't easily fit on a single server, Instant Clusters can also be used for deployment—giving you the flexibility to serve massive models at scale when serverless isn't a fit. While most production workloads benefit from serverless, clusters are a powerful option for specialized deployment needs.
By offering both clusters and serverless compute, RunPod helps AI teams move faster, control costs, and match their infrastructure to their real-world workflows—no matter where they are in the development lifecycle.
Final Thoughts
Scaling AI isn't just about "going bigger."
It's about choosing the right infrastructure for the right task.
GPU clusters give you the power to train and fine-tune complex models efficiently. Serverless GPUs give you the speed and flexibility to deploy those models to the world.
At RunPod, we're here to help you do both—and to do it better.