Four Reasons To Set Up A Network Volume In the RunPod Secure Cloud
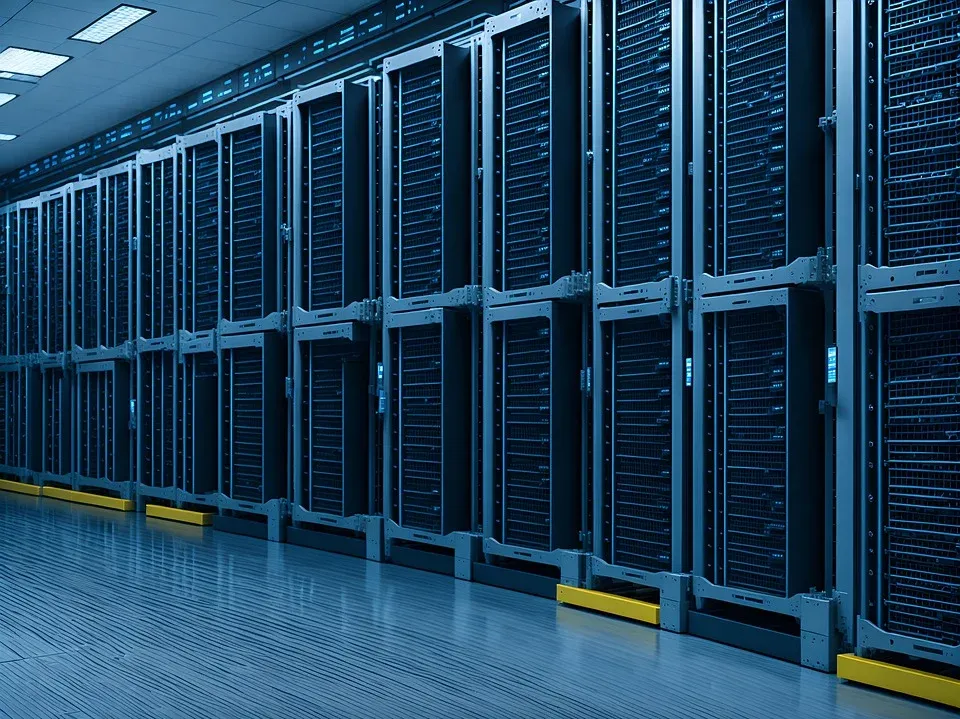
Network Storage is a new RunPod feature (currently in beta) that works with our Secure Cloud data center-hosted pods. Normally, volumes for pods are destroyed irrecoverably after you terminate the pod, but this allows storage to persist and even be reassigned to different pods if needed. Here are several reasons why you might find this useful.
How Network Storage Works
Network Storage creates a persistent volume that exists independently of any individual pod. This volume is hosted in RunPod's secure data centers and can be attached to any compatible pod in the same data center. The data on these volumes persists even when all pods using it are terminated, allowing you to maintain your work environment across sessions.
Key technical aspects:
- Storage is provided through a high-performance network file system
- Volumes can be accessed simultaneously by multiple pods
- Data is stored redundantly to protect against hardware failures
- Access is secured through RunPod's authentication systems
- Available in all RunPod Secure Cloud data centers
Here are some of the most compelling reasons to use network volumes:
1.) Allows you to run multiple pods with the same data
One of the handiest reasons is it allows you to use the same data on multiple machines. Say you're training or finetuning a model - that's going to take up the entire GPU's resources, and you're not really going to be able to easily stop training and just test out the model without potentially cooking or corrupting the model. You could transfer the data from one pod to another to test it out and see how it is shaping up with runpodctl, but honestly there's a better way.
All you need to do is set up two pods that reference the same network volume, and both pods will be able to see and act on the data. You could let your first pod continue to train, and point your second pod running, say, ComfyUI to the same folder that the model checkpoints are appearing and easily test them out as they come out.
This flexibility is particularly valuable for:
- Handling fluctuating availability of specific GPU types
- Scaling up to more powerful GPUs when needed for larger workloads
- Scaling down to more cost-effective GPUs for less intensive tasks
- Transitioning between development and production environments
2.) Greater flexibility to move between pod types
If you are running very computationally demanding tasks, you may need to be more selective and use higher-end pods which may also be in higher demand by other users. For example, if you need an RTX 6000 or comparable to run your task, but none of the 6000s are available, you can simply just pick up and move your workspace to an A100 or L40 with a minimum of effort rather than having to start from scratch.
3.) Save money on disk space by assigning a network volume to multiple pods
If you need to run multiple pods simultaneously, you can achieve significant cost savings by assigning the same network volume to all of them, rather than provisioning separate storage for each pod. This eliminates duplicate data and reduces your overall storage costs. Remember, you're billed separately for the volumes you use on each pod.
For teams working on the same project, this shared storage approach also simplifies collaboration and ensures everyone is working with the same datasets, models, and code.
4.) Keep your work saved in a secure data center as opposed to on your local drive
If you're a visual artist in AI or any other discipline that requires a lot of iteration, you may be tempted to keep your work stored on your local drive which may leave it vulnerable to data loss (if your hard drive crashes, for example.) Keeping your data stored in a network drive in the data center means that it will be saved in a secure location with redundancy and data backups rather than leaving you at the mercy of crashes, power outages, and other environmental concerns.
Other Practical Use Cases
Collaborative AI Development
Teams can work simultaneously on the same project data. One team member might be preparing datasets while another is training models and a third is evaluating results - all accessing the same storage volume but with different pod configurations optimized for their specific tasks.
Continuous Training with Periodic Evaluation
Set up a continuous training pipeline where one pod handles the long-running training process while separate, shorter-lived pods can spin up periodically to evaluate the latest model checkpoints, generate test outputs, or run benchmark tests.
Multi-Stage ML Pipelines
Create complex machine learning pipelines where different stages of the workflow (data preprocessing, training, evaluation, inference) can be handled by specialized pods, all reading from and writing to the same network volume to maintain workflow continuity.
Seamless Development-to-Production Transition
Develop and test your AI models on lower-cost GPUs, then seamlessly transition to production-grade hardware for final training or deployment without needing to migrate your entire workspace.
Questions about creating a network volume?
We have a guide to walk you through creating a network volume in our Readme – if you have any further questions, feel free to reach out directly!