5090s Are Almost Here: How Do They Shape Up Against the 4090?
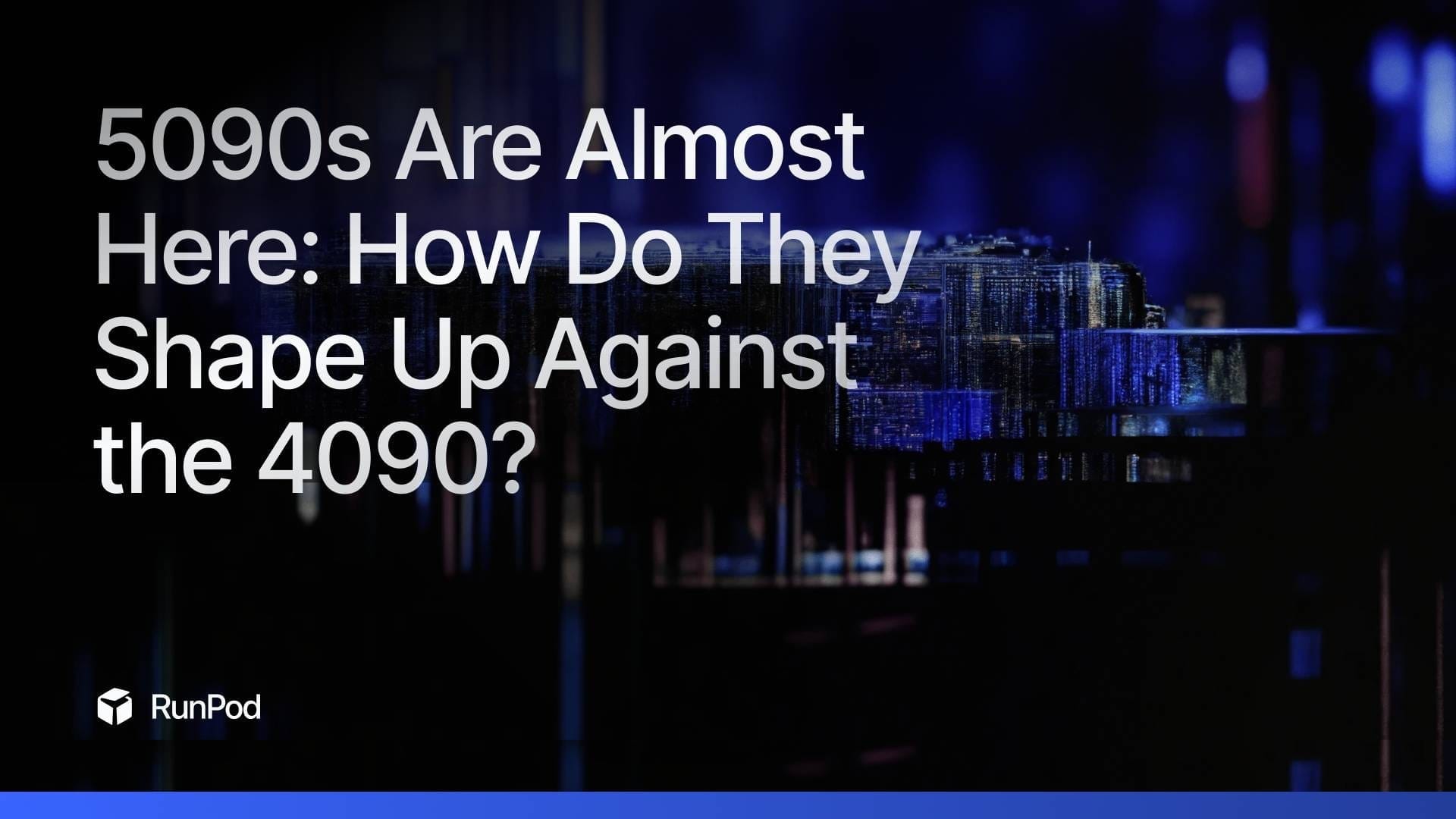
Another year has come and another new card generation from NVidia is on the way. 5090s are due to become widely available this January, and RunPod is going to be extremely eager to support them once they do. Along with the new Blackwell architecture, the 5090 is set to launch with 32GB of VRAM, one of the most exciting memory upgrades since we saw the H200 launch with 141GB. More memory on a single card is always exciting, as it significantly expands use cases for applications, especially those without great parallelism support.
Hard Stats
Here are how the specs between the two cards shake up (courtesy NVidia's official spec sheet)
RTX 5090 | RTX 4090 | |
---|---|---|
Architecture | Blackwell | Ada |
AI TOPS | 3352 | 1351 |
Memory | 32GB GDDR7 | 24GB GDDR6X |
Memory Bandwidth | 1792 GB/S | 1008 GB/S |
CUDA Cores | 21760 | 16000 |
Streaming Microprocessors | 170 | 128 |
Ray Tracing Cores | 170 | 128 |
Tensor Cores | 680 | 512 |
But how do these stats shake out when the rubber meets the road? Early performance metrics conducted through OpenCL and Vulkan report a 16% and a 37% increase over the 4090, respectively.
NVidia has also released its own stats on how the 5090 shapes up over the 4090 in gaming performance. Although our GPUs obviously aren't used in this environment, it is still worth sharing this information as a bellwether for the kind of overall performance jump that might be expected:
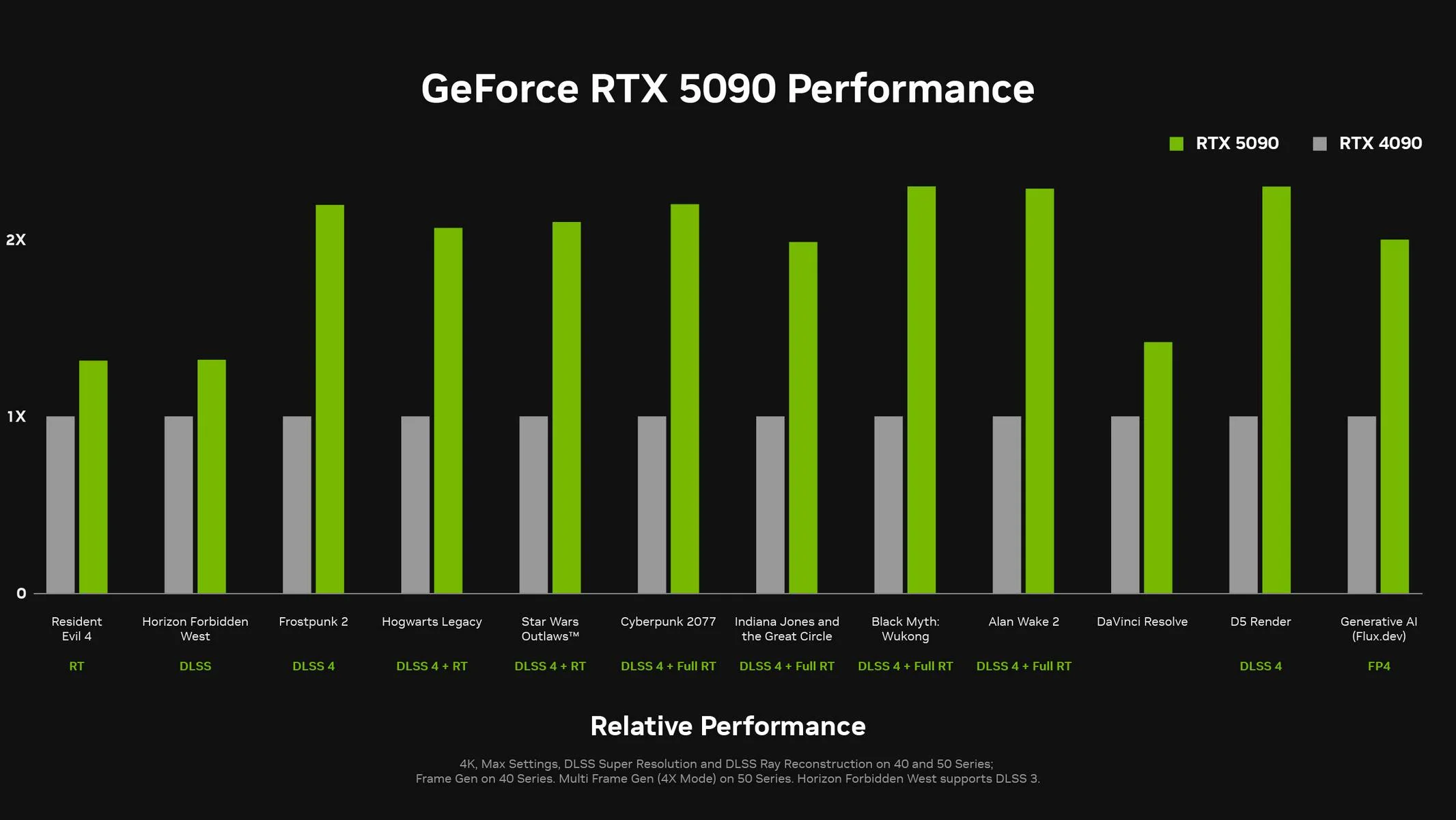
Let's break down how these improvements benefit you.
For AI/ML Workloads:
- The 148% increase in AI TOPS (3352 vs 1351) represents a massive leap in AI inference and training capabilities. Clients running large language models, computer vision systems, or other AI applications should see significantly faster training times and inference speeds.
- The increased Tensor Core count (680 vs 512, ~33% more) coupled with the architectural improvements of Blackwell will particularly benefit matrix multiplication operations, which are fundamental to deep learning.
Memory Advantages:
- The 33% increase in memory (32GB vs 24GB) allows handling of larger AI models and bigger batch sizes in a single pass.
- The substantially higher memory bandwidth (1792 GB/s vs 1008 GB/s, ~78% increase) means faster data loading and less time spent waiting for memory transfers.
- GDDR7 vs GDDR6X technology suggests better power efficiency per bit transferred.
- The 148% increase in AI computation power means you can expect to see training times nearly cut in half for many common ML tasks. For example, fine-tuning a medium-sized language model (7B parameters) on a custom dataset that previously took 24 hours could now potentially complete in approximately 10-12 hours. As the pricing for the 5090 will be competitively priced to existing hardware this will almost certainly result in a bottom line savings per minute of GPU time.
- The increased memory bandwidth (1792 GB/s) particularly benefits training scenarios with large batch sizes, allowing for more stable and efficient training of computer vision models and transformers.
General Compute Benefits:
- The 36% increase in CUDA cores (21760 vs 16000) will benefit any parallel processing workloads.
- More Streaming Multiprocessors (170 vs 128, ~33% increase) means better concurrent task handling and workload distribution.
When Are 5090s Coming?
Soon (tm). It's hard to announce specifics but we are already talking to our data center and cloud partners and they are well aware of the demand. We are committed to keeping the pricing competitive and reasonable to put this expensive hardware in the hands of even the smallest developer teams.
If you're interested, sign up for our interest form here and you'll be the first to know when they go live - in the meantime, keep watching our blog and our Discord for updates!